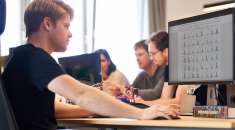
#data #data science #data thinking
25. Juni 2019 |
- min Lesezeit
Imagine the following situation:
Can you relate to that situation? Have you ever experienced this kind of situation? At least you are not alone. According to Gartner 87% have low business intelligence and analytics maturity.
Basically, there are two different modes in product management.
This article focuses on the operate mode.
In order to streamline the operation mode of your product with data you can follow these steps.
Data that is created when your users interact with you product are relevant. Most of the time it stems from web analytics systems, but other data sources are relevant as well. For example in ecommerce the return rate is pretty important but is usually not contained in your web analytics data. Make sure your data collection layer is reliable and flexible as changing requirements are guaranteed once you work intensively with your data. For example a tag management system nowadays is a must. (LINK?) But how to determine which data is relevant? Start by defining your KPIs and metrics. They should make up a coherent system from raw data over metrics to KPIs. See this article (in German) for more information about KPIs.
Everybody has dashboards and the usual tools even provide pre-defined ones. However, rarely have I seen a reasonable, useful and well designed dashboard that helps product managers in their daily work. Dashboards are made to inform you if everything is fine or not – they raise the question but do not provide the answer. When designing dashboard (the overview) and reports (detailed information to look at if something unexpected happened) always work iteratively and make heavy use of prototyping. An important aspect is the integration of and comparison with target figures. Easy with BI tools and custom data platforms, complicated with web analytics tools.
Once you set up your dashboards (ranging from basic ones as a dashboard to show you the conversion funnel per marketing channel to more advanced as a dashboard to show the adoption and retention rate of a new feature/product) and you see a KPI drop significantly, you should dig into your data in order to find the root cause. You start with your predefined reports but if they aren’t sufficient use raw data and custom reports in order to identify the root cause of the changes in your numbers. Doing this regularly gives you a deep understanding of your product and the underlying mechanics.
Nowadays all relevant web analytic tools provide the ability to create alerts. Some even provide automatic recognition of unusual events (anomaly detection). But no one should know your data better than you. You should have a clear understanding of your target numbers and set up means to inform you whenever reality significantly misses your expectations. This releases you from manually checking your data every now and then and let you focus on more important tasks.
This is important. All your efforts to intensively work with data will fail if the company you are working in doesn’t appreciate that. From the beginning on use and show your data. Whenever you present you work and future plans show the data you used for decision making. Instead of gut feelings argue with reports and data charts. Whenever you do stakeholder management have the data based insights ready. Show them how effortless you work with data and what quality improvement this means to your decisions and of course what successes you have by using data. This will help to establish a data driven culture in your company.
Whenever you plan to improve your product look into your data. Check your highest exit rates, common fraud patterns or worst selling products. Come up with hypothesis for the underlying causes and how you can change that. Maybe you even find examples in your data that clearly validate your hypothesis. List all your hypothesis with references in the underlying data exploration in a hypothesis backlog.
Once you have a hypothesis it is time to test it. Depending on the hypothesis you would choose the appropriate method to validate it. (This alone would make a blog post of its own). If you can apply A/B or multivariate testing you get a new set of data and arguments for data-driven decision making that is difficult to argue about.
This is only a small overview about how to utilize data for your daily work with an established product. The bottom line is that you have to start somewhere and then continually improve. The basic idea is to follow the same pattern regardless of how advanced your data usage is: start by manually exploring your data, get insights, know and understand your data. Once you reached the next level of understanding try to automize your manual work in order to free your resources mentally and time-wise for the next topics. In the next article I will write about the right part of the Gartner data maturity model and how to use data for the product management mode innovation.