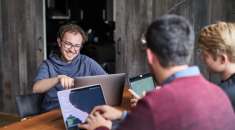
#data #databased #datascience
21. Okt. 2019 |
- min Lesezeit
Workshops and coachings are an important part of our daily work and mission, as we help customers and partners to develop a lean, user-centered mindset that enables the development of successful, digital products.
Since the inception of their activity almost three years ago, we share our experience with the Digital Product School (DPS) by unternehmerTUM. In a three-months program students and company partners are offered the possibility to learn hands-on how a cross-functional team builds a digital product, from the discovery phase to the actual development of an MVP. So far, our main contribution to the program was related to Design Thinking, Lean Startup and Stakeholder Management, and after a few interesting conversations we realised that our visions concerning the role of Data and AI in product development were convergent, so that we extended our collaboration in this direction.
It is not a secret that we are promoting the development of a “data culture” in companies as a prerequisite for any successful application of AI. A data-driven business should include the data perspective in all phases of product development, together of course with the users.
In our “Innovate with Data” workshop we focused on the first two steps, helping the eight teams of the DPS to incorporate data in their thinking of the problem space and product ideas. They arrived with a sound understanding of their users pain points, and left with inspiring ideas on how to innovate with data. We structured our workshop in three parts, each with its significant takeaways.
“
"Data culture" is a prerequisite for any successful application of AI
“
In the first part, we helped the teams to list data related to their problem space. With the help of the Data Landscape Canvas by Datentreiber the participants could identify and classify relevant existing data sources, as well as be creative about possible ways to generate data with their products. At this stage one can also include, for example, publicly available data that are not directly related to the addressed problem, but might be helpful in prototyping and validating assumptions (traffic data from different cities, or different target groups with similar pain points etc.).
You need to get to know your data before doing something with it. Your dataset - typically a table with lots of numbers and other stuff - contains precious information that might help you in two directions: on one side you might look for quantitative validation of the hypotheses and assumptions that emerged during your preliminary qualitative research (interviews etc.); on the other, interesting unexpected insights might arise that suggest new ways to generate value for your users. The technical ability to analyse the data is important, but useless without a deep understanding of the business side of the problem. That’s why at this stage we encourage a strong collaboration between the data scientist and the product manager: the latter can ask the right questions, which the former can answer by digging into the data. For the workshop we didn’t want to focus on tools, rather on best practices: we showed an example of EDA performed on the NY Citibike dataset, where we discovered many interesting things, like the commuting pattern shown in the image below. EDA can offer many useful insights, and you could spend weeks exploring the hidden beauty of your data; you will eventually need to stop, but always keep in mind that an incomplete EDA is always better than no EDA!
“
Product managers can ask the right questions, which data scientists can answer by digging into the data.
“
Creativity is a defining element of being a “ProduktMacher”, and we have a great deal of experience with different ideation techniques, from guided brainstormings to Crazy Eights and so on. What about data products, though? How to give everyone in a product team the possibility to fantasize on the possible though realistic applications of AI to their specific problem? The answer is Aideate, our AI-cards for guided brainstorming. The idea came from Doblin’s Ten Types of Innovation, with a focus on AI use-cases: we defined five macro-categories - Computer Vision, Natural Language Processing, Audio Processing, Recommendations and Predictive Intelligence - each associated to a number of cards describing the more specific AI techniques (like Object Detection, Predictive Maintenance and so on).
For each technique we showed to the participants a card containing a short high-level description, and gave them two minutes to discuss its possible applications to their specific problem / product. After almost two hours of intense creativity everyone had discovered previously unimaginable ways to generate user value by adding Sentiment Analysis, Image generation, or Network Analysis to their product, coming up with ideas that might strongly contribute to their UVP.
We presented our protocol for data-driven innovation in its three steps:
We now look forward to observing how this will impact the products that the teams of Digital Product School are going to build in the next few weeks!